When describing the potential benefits of an Industrial Internet of Things solution, we can begin at the basic level of sensor implementation and then discuss how the impact grows as the scope expands and the data becomes more significant to the business. Increasing integration with systems and business processes optimizes the value, particularly when technology choices easily enable interfaces and support scaling without a substantial increase in cost. An excellent way to illustrate this is with an IoT example in a manufacturing environment.
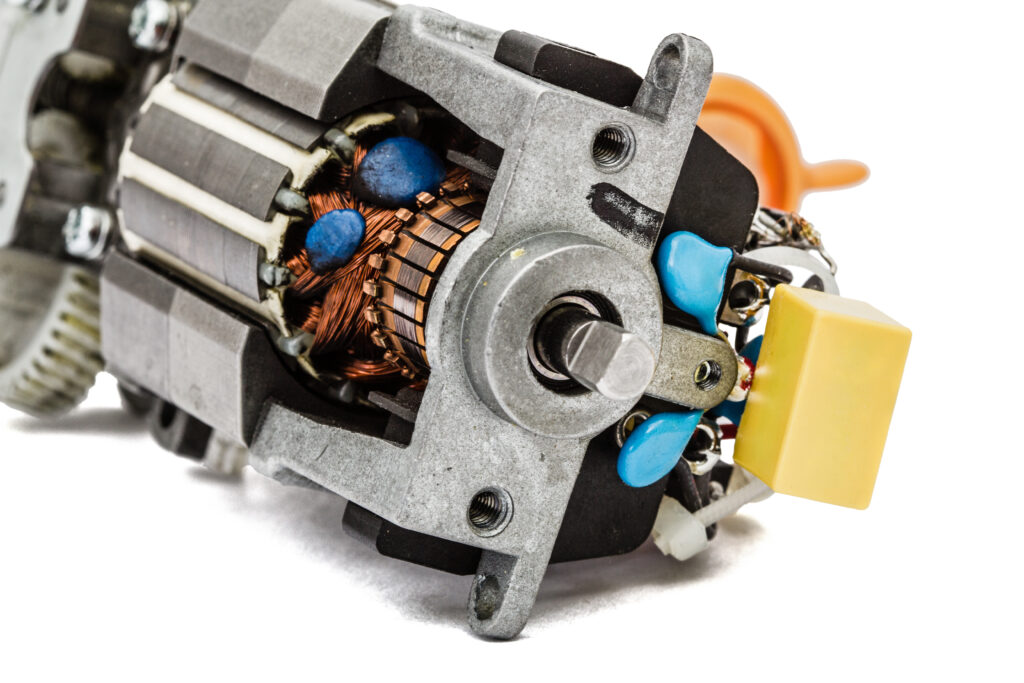
We can start by adding a vibration and temperature sensor to a single electric motor in a factory. For each of these attributes, we can get normal readings from specifications, previous experience, or an initial monitoring period with our new sensors (assuming the chosen motor is functioning correctly).
Assuming the project will grow in scope, we need to choose an IoT application environment to ingest, store, and visualize the data. Robust device management is also essential.
The data from the sensors is tracked to look for deviations from the norms. Deviations from acceptable values create alerts. And, if the deviation is critical, a notification is sent to the technician responsible for the unit’s performance and maintenance. The notifications and supporting processes are fine-tuned, eventually providing input to a preliminary cost-benefit analysis, which should drive a decision to increase the scale of the project.
Assuming the choice is made to grow the project, adding the same sensing capabilities to multiple motors of the same type makes sense, providing more data for analysis. Norms, significant deviations, and alerts are adjusted based on this more extensive data universe. Maintenance processes undergo additional refinement with additional experience.
We now have a wealth of information for assessing equipment performance and maintenance processes. It’s time to evaluate the effect on personnel expense, the incidence of machine failure, increased output, etc.
Now, let’s assume this particular motor type is sourced from two different manufacturers serving a similar purpose but having some slight differences in design. Separating the data between the two types can deliver a wealth of information. If the product from one of the manufacturers has a shorter useful life, it’s probably already known. But what if this product shows vibration and temperature reading deviations much earlier, possibly during the warranty period? From a customer perspective, there could be a possibility of getting a warranty replacement at no charge versus recording a loss a few months later. We also have an opportunity to communicate our findings to the manufacturer, perhaps spurring them to reevaluate their product.
Stepping it up by incorporating machine learning (AI/ML) enables the identification of significant patterns in the data. This automated analysis of large amounts of data could show how running motors at different speeds impacts their performance and durability. As motors age, data could be fed into an assessment of the entire equipment fleet, broken down by predicted remaining operational life for inventory, ordering, and financial processes.
Machine learning could be further leveraged to have a greater impact on the costs and efficiency of the business. The model describing the effect of various speeds on the performance and expected useful life of motors could be input to an actuating process that controls the speed of motors to ensure they perform efficiently. Another example could be a machine learning algorithm assessing the condition of all motors across the enterprise and, based on inventory, projecting the number of units that need to be replaced over time, automatically creating orders for new ones. Human approval of these orders would probably be advisable, but this could be another way to increase efficiency and lower the total cost of ownership (TCO).
The success of an implementation like the one described requires good planning and execution. An important aspect is making the best technology decisions early, particularly in the selection of a software platform that enables a low-cost rapid deployment of a Proof of Concept, facilitates an efficient transition to a large-scale implementation, and provides interfaces (“APIs”) to other applications such as Machine Learning capabilities, ERP, and manufacturing control systems.